
The suggested ternary neural network with a complementary binary array for signed synaptic weights may save up to 70% of the memory needed for the model's parameters. The recognition rate for voice instructions was 89 percent. The suggested ternary neural network recognized same dataset objects in real-time, the identification percentage for recorded items was 87%. We created our own customized dataset consisting of 3750 images of objects to train and test the proposed neural network for object recognition. To improve the efficiency of the neural network, the multiplication of two binary values is replaced with the bitwise AND operation. Signed synaptic weights were represented using a complementary binary array to save memory. Signed synaptic weights of-1, 0, and 1 are represented as 8-bit integers in neural networks. To recognize voice and images, the suggested ternary neural network is integrated on a Raspberry Pi board. more The signed synaptic weights are represented using a ternary neural network with complementary binary arrays. The signed synaptic weights are represented using a ternary neural network with complementary bin. The suggested ternary neural network with a complementary binary array for signed.
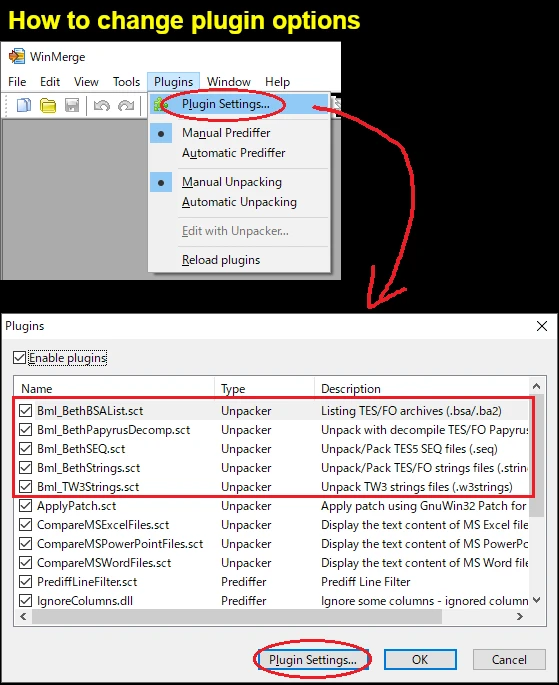
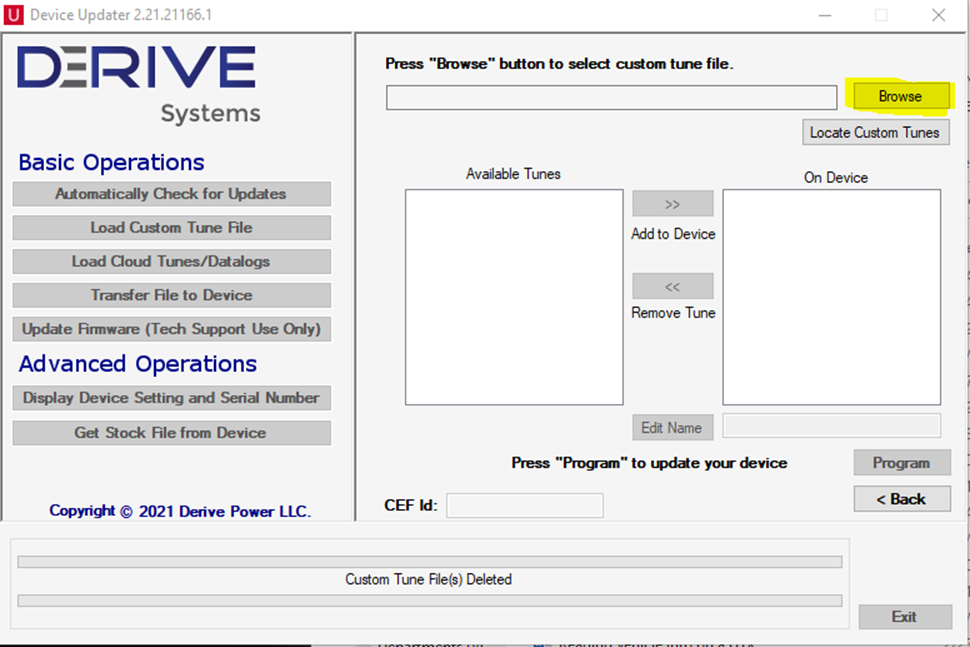
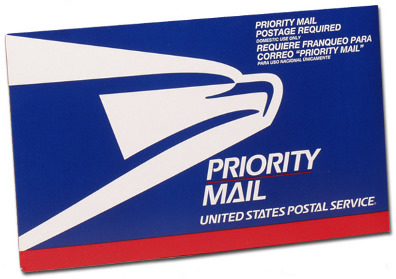
Signed synaptic weights of –1, 0, and 1 are represented as 8-bit integers in neural networks.
